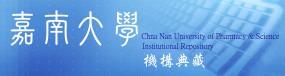 |
English
|
正體中文
|
简体中文
|
全文筆數/總筆數 : 18263/20495 (89%)
造訪人次 : 7021243
線上人數 : 1567
|
|
|
資料載入中.....
|
請使用永久網址來引用或連結此文件:
https://ir.cnu.edu.tw/handle/310902800/34626
|
標題: | Graphite Classification of Gray Cast Iron in Metallographic via A Deep Learning Approach |
作者: | Huang, Wesley Su, Zhi-Yuan Wang, Chia-Sui Yeh, Mark Chou, Jyh-Horng |
貢獻者: | Department of Information Management, Chia Nan University of Pharmacy & Science Department of Multimedia and Game Development, Chia Nan University of Pharmacy & Science National Sun Yat Sen University Feng Chia University |
關鍵字: | neural-network yolo |
日期: | 2022 |
上傳時間: | 2023-12-11 14:01:22 (UTC+8) |
出版者: | LIBRARY & INFORMATION CENTER, NAT DONG HWA UNIV |
摘要: | In addition to measurements of physical and mechanical properties, quality inspections also include metallographic analyses. When gray casting iron material, different manufacturing processes cause different microstructures in the material, whose metallographic images also perform large differences. The metallographic properties of gray iron can be divided into six types (from Type A to Type F). The proportion of types will influence the strength, wear resistance, and lifetime of specimens. The determination of type is usually dependent on manual judgments. In this study, two approaches were developed to analyze six metallographic types of gray casting iron. The first approach was to determine the type according to features of the detected particles in the metallographic materials by morphology algorithm. Types A, C, and F could be identified with the shape factor (SF) of gray casting iron. Then, the remained part could be identified using average grayscale values of the part-region of the metallographic material. Second approach was to identify Types A, C, and F with SF method and then identify the remaining part through the classification of the YOLO V3 deep learning algorithm. The results showed that the second approach performed more suitably in identifying the types of metallographic of gray casting iron. |
關聯: | Journal of Internet Technology, v.23, n.4, pp.889-895 |
顯示於類別: | [資訊管理系] 期刊論文 [多媒體與遊戲發展系] 期刊論文
|
文件中的檔案:
檔案 |
描述 |
大小 | 格式 | 瀏覽次數 |
2740-3730-1-PB.pdf | | 667Kb | Adobe PDF | 151 | 檢視/開啟 | index.html | | 0Kb | HTML | 240 | 檢視/開啟 |
|
在CNU IR中所有的資料項目都受到原著作權保護.
|