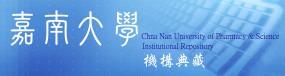 |
English
|
正體中文
|
简体中文
|
全文筆數/總筆數 : 18034/20233 (89%)
造訪人次 : 23776927
線上人數 : 767
|
|
|
資料載入中.....
|
請使用永久網址來引用或連結此文件:
https://ir.cnu.edu.tw/handle/310902800/34430
|
標題: | Predicting the 14-Day Hospital Readmission of Patients with Pneumonia Using Artificial Neural Networks (ANN) |
作者: | Tey, Shu-Farn Liu, Chung-Feng Chien, Tsair-Wei Hsu, Chin-Wei Chan, Kun-Chen Chen, Chia-Jung Cheng, Tain-Junn Wu, Wen-Shiann |
貢獻者: | Chi Mei Med Ctr, Pulm Med Chi Mei Med Ctr, Dept Med Res Chi Mei Med Ctr, Dept Pharm Chi Mei Med Ctr, Div Clin Pathol Chi Mei Med Ctr, Dept Informat Syst Chi Mei Med Ctr, Dept Neurol Chi Mei Med Ctr, Dept Occupat Med Chi Mei Med Ctr, Div Cardiovasc Med Chia Nan Univ Pharm & Sci, Dept Pharm |
關鍵字: | unplanned patient readmission artificial neural network convolutional neural network nurse Microsoft Excel receiver operating characteristic curve |
日期: | 2021 |
上傳時間: | 2023-11-11 11:52:21 (UTC+8) |
出版者: | MDPI |
摘要: | Unplanned patient readmission (UPRA) is frequent and costly in healthcare settings. No indicators during hospitalization have been suggested to clinicians as useful for identifying patients at high risk of UPRA. This study aimed to create a prediction model for the early detection of 14-day UPRA of patients with pneumonia. We downloaded the data of patients with pneumonia as the primary disease (e.g., ICD-10:J12*-J18*) at three hospitals in Taiwan from 2016 to 2018. A total of 21,892 cases (1208 (6%) for UPRA) were collected. Two models, namely, artificial neural network (ANN) and convolutional neural network (CNN), were compared using the training (n = 15,324; approximately equal to 70%) and test (n = 6568; approximately equal to 30%) sets to verify the model accuracy. An app was developed for the prediction and classification of UPRA. We observed that (i) the 17 feature variables extracted in this study yielded a high area under the receiver operating characteristic curve of 0.75 using the ANN model and that (ii) the ANN exhibited better AUC (0.73) than the CNN (0.50), and (iii) a ready and available app for predicting UHA was developed. The app could help clinicians predict UPRA of patients with pneumonia at an early stage and enable them to formulate preparedness plans near or after patient discharge from hospitalization. |
關聯: | INT J ENV RES PUB HE, v.18, n.10 |
顯示於類別: | [藥學系(所)] 期刊論文
|
文件中的檔案:
檔案 |
描述 |
大小 | 格式 | 瀏覽次數 |
ijerph18105110.pdf | | 6495Kb | Adobe PDF | 49 | 檢視/開啟 | index.html | | 0Kb | HTML | 71 | 檢視/開啟 |
|
在CNU IR中所有的資料項目都受到原著作權保護.
|